Decomposing complex traits through crop modelling to support cultivar recommendation. A proof of concept with a focus on phenology and field pea
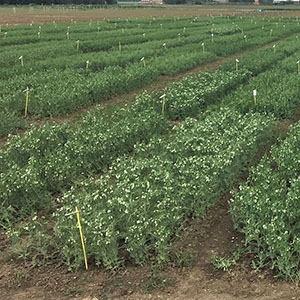
Accepted: 7 January 2022
Appendix: 59
HTML: 9
All claims expressed in this article are solely those of the authors and do not necessarily represent those of their affiliated organizations, or those of the publisher, the editors and the reviewers. Any product that may be evaluated in this article or claim that may be made by its manufacturer is not guaranteed or endorsed by the publisher.
Cultivar recommendation is crucial for achieving high and stable yields, and crop models can successfully support it because of their capability of exploring genotype environment management interactions. Different modelling approaches have been developed to this end, primarily relying on dedicated field trials to characterize the germplasm of interest. Here, we show how even data routinely collected in operational contexts can be used for model-based cultivar recommendation, with a case study on phenological traits and field pea (Pisum sativum L.). Eight hundred and four datasets, including days from sowing to plant emergence, first flower, and maturity, were collected in Northern Italy from 2017 to 2020, and they were used to optimise six parameters (base, optimum, and maximum temperature for development, growing degree days to reach emergence, flowering, and maturity) of the crop model WOFOST-GT2 for 13 cultivars. This allowed obtaining the phenotypic profiles for these cultivars at the level of the functional trait, without the need of carrying out dedicated phenotypisations. Sensitivity analysis (SA) techniques (E-FAST) and the statistical distributions of the optimised parameters were used to design pea ideotypes able to maximise yields and yield stability in 24 agro-climatic contexts (three soil conditions two sowing times four agro-climatic classes). For each context, the 13 cultivars were ranked according to their similarity to the ideotype based on the weighted Euclidean distance. Results of SA identified growing degree days to reach flowering as the trait mainly affecting crop productivity, although cardinal temperatures also played a role, especially in the case of early sowings. This is reflected in the ideotypes and, therefore, in cultivar ranking, leading to recommend a panel of cultivars characterised by low base temperature and high thermal requirements to reach flowering. Despite the limits of the study, which is focused only on phenological traits, it represents an extension of available approaches for model-aided cultivar recommendation, given that the methodology we propose can take full advantage of the potentialities of crop models without requiring dedicated experiments aimed at profiling the germplasm of interest at the level of functional traits.
Highlights
- Crop models are powerful tools to support cultivar choice by exploring genotype x environment x management interactions.
- Crop models require cultivar-specific phenotyping data at the level of functional traits.
- We propose a methodology that uses data routinely collected in operational contexts to derive functional trait values.
- This study is a proof of concept of how to increase the applicability of model-based approaches for cultivar choice.
How to Cite

This work is licensed under a Creative Commons Attribution-NonCommercial 4.0 International License.
PAGEPress has chosen to apply the Creative Commons Attribution NonCommercial 4.0 International License (CC BY-NC 4.0) to all manuscripts to be published.